eCommerce Product Recommendations: Benefits, Examples, Strategies & Types of eCommerce Recommendation Systems to Increase Online Sales [2024]

Naturally, technology is something that has an innate ability to scale up, by feature. These days, and especially so in the dynamic world of eCommerce, staying ahead of the competition means making the most of every resource available to you. Your brand needs to level and adapt with the market tides if you don’t want to get drowned out by your competition.
By far, including product recommendation systems for eCommerce, into the online shopping experience for customers is a method that is frequently overlooked and underutilized. In eCommerce, personalization not only makes for a better shopping experience, but it also plays an important role in increasing sales and cultivating customer loyalty to your brand. And eCommerce product recommendations are the most impactful way of accomplishing this.
In this comprehensive article, we’ve explored a lot of questions: What are eCommerce Product Recommendations, at the core of it? How can they benefit your business? What are the various types of eCommerce recommendation systems and the best strategies to leverage them? How can your brand incorporate a robust product recommendation system for eCommerce in a way that feels genuine to your customer and not too gimmicky? Read on to find out.
What Are eCommerce Product Recommendations?
eCommerce product recommendations refer to the customized recommendations that are offered to customers depending on their past actions, such as their shopping history.
In most cases, these recommendations are generally pushed through product banners across a website, or emails, or within the critical sections of the mobile app.
In its essence, eCommerce product recommendations are a digital substitute for an informative salesperson who is familiar with a customer’s preferences and requirements. It employs information and computerized techniques to identify which products the customer is likely to purchase next, making the shopping process more personalized and time efficient.
Here’s how eCommerce Product Recommendations benefit sales and retention in online retail:
- Personalized Shopping Experience: Recommendations make every customer’s experience individualized, to fit his or her wants and desires.
- Increased Sales: In this way, businesses can increase cross-sell and up-sell rates and have a direct influence on the company’s profit margin.
- Customer Engagement: Relevant recommendations maintain the customers’ attention on the site for a longer time and thus there are high chances of making a purchase.
- Improved Product Discovery: Recommendations assist the customers to find products they could not have otherwise come across, thus increasing their awareness of your catalog.
- Reduced Decision Fatigue: Recommendations assist in reducing large varieties of products, and this makes the decision-making process easier for the customers.
- Data-Driven Insights: Recommendation interactions produce data insights, that are useful in understanding your customers’ behavior and their preferences.
Through the use of eCommerce product recommendations, businesses can be able to design an ever-responsive, flexible, and lucrative online shopping experience for the benefit of the business and the buyer.

Benefits of eCommerce Product Recommendations
Customers are influenced to buy more as the products recommended to them are likely to be what they need. Thus, when businesses promote products that complement others, they can easily influence the average order value through cross selling and upselling. For example, Amazon has credited as much as 35% of its total sales to its eCommerce recommendation system. This strategy not only promotes the sale of the product in the current instance but also makes the customer aware of other products, thereby increasing the chances of future business and hence, customer lifetime value.
Enhanced User Experience
eCommerce Recommendation systems improve the efficiency of the shopping process and customer satisfaction when searching for a specific item or browsing through the catalog. This makes the search process much faster and less tiring for the consumer especially in stores with large product offers. Recommendations make the list easier to browse through as they offer the relevant options and suggestions, which is like having a personal shopper. This improved user experience tends to result in higher levels of customer satisfaction, longer time spent on the website, and better conversion rates.
Improved Customer Loyalty
Thus, with the help of the offered personalized and relevant products, eCommerce sites can build a better relationship with their customers. Such a strategy makes the shoppers feel valued, and thus they are likely to shop again in the future. Good recommendations show that a business entity comprehends its clients’ tastes and requirements, and this creates confidence. This over time changes the one-time customers into repeat customers and brand promoters, thus enhancing the customer lifetime value.
Optimized Marketing Spend
eCommerce Recommendation systems help to save time on marketing and be more specific. Thus, using customer data and behavior analysis, organizations can target specific products that are most likely to generate customer interest. This approach eliminates the spending of large amounts of money in advertising that will not be of any value to the business, thus increasing the value of the marketing strategies. Also, appropriate on-site suggestions can reduce the need for expensive off-site promotion as the targeted products will be promoted within the platform.
Data Insights for Continuous Improvement
eCommerce Recommendation systems gather useful information about customers, their buying behavior, and products. These are not mere sales data but rather give deeper analysis of the customers and the market. This information can then be used by the businesses to make decisions concerning the inventories, products and the overall business. Through recommendation data, companies are able to predict trends, view the relationships between products, and make ongoing improvements to products and marketing strategies to cater to the customer’s needs and the market.
Types of eCommerce Recommendation Systems in 2024
Collaborative Filtering Systems
Collaborative filtering systems use the information of other users to recommend products. This approach involves the use of historical data in the use of products by customers, their purchase patterns, and their ratings. There are two main types: The two types of recommendations are user-based (items that users with similar preferences have bought) and item-based (items that are usually purchased together). Despite its efficiency for well-developed products, collaborative filtering can perform badly with new goods or users because of the “cold-start” issue. However, this invention still persists to this date because of its uniqueness that it offers surprising recommendations.
Content-Based Filtering Systems
Content-based filtering recommends products by analyzing the attributes of items a user has previously shown interest in. This system creates a profile for each product and user, matching them based on similarities. For instance, if a user frequently purchases science fiction books, the system might recommend other sci-fi titles. Content-based filtering excels at providing highly relevant recommendations and works well for niche products. However, it may limit discovery by continually suggesting similar items, potentially creating a "filter bubble" effect.
Hybrid Recommendation Systems
Hybrid systems incorporate more than one recommendation technique so that the benefits of each method can be obtained while the drawbacks of each method are avoided. For instance, it may use the collaborative filtering method to offer diverse recommendations and the content-based filtering method to offer relevant recommendations. This approach can solve the problems that are inherent to the single-method systems, for instance, the cold start problem or over-specialization. Hybrid systems provide better and precise suggestions since they are flexible to different situations and users’ actions.
AI-powered Recommendation Systems
eCommerce Recommendation systems using AI in e-commerce are the pinnacle of personalization technologies in 2024. These systems employ machine learning, deep learning and natural language processing to process huge volumes of information and deliver accurate contextualized recommendations. AI systems can take into account the factors such as seasonality, current trends, and even the user’s subtle signals. They never stop learning and getting better and their accuracy increases with time. AI-powered product recommendation systems for eCommerce, like App0, take the overall eCommerce personalization customer experience to a whole new level.
Effective Strategies For Your eCommerce Recommendation System in 2024
Personalization Techniques
Try to use user data, browsing history, and purchase patterns to make dynamic personalization. Employ AI algorithms to study the customers’ preferences and make the recommendations unique. Take into account such factors as time of day, season, and location of the business. Use to tailor the product offerings, search results and promotional emails. It is necessary to apply the user segmentation to form the recommendation groups. Update the user’s information and preferences from the interactions, and feed them to the personalization algorithms to increase their efficiency in the subsequent interactions.
Utilizing Customer Data
Gather multiple kinds of information such as customers’ previous orders, recent visits, items added to favorites, and feedback on specific products. It is recommended to utilize this data to make detailed user profiles. Use data mining to find out underlying patterns and preferences. Data privacy compliance and getting the most out of the data. Connect data from the mobile application and in-store buying activity for better understanding of the customer’s behavior. Ensure that you update and clean your data as often as possible to ensure that you are working with the right data.
A/B Testing and Optimization
Experiment with new recommendation algorithms, its placement, and design to improve its efficiency. Employ A/B testing to determine which variation yields the best results to apply the same to the strategies. Experiment with parameters such as the number of items recommended, format of the recommendations and time of the recommendation. Evaluate the click-through rates, the conversion rates of the links, and the average order value. A strategy that should be adopted is the systematic procedure of testing where only one factor is changed at a time. Employ the information obtained to improve your eCommerce recommendation system in successive steps.
Integrating with Marketing Campaigns
The recommendation strategies should be aligned with other marketing activities to ensure a consistent customer experience. Feature product recommendations in emails, remarketing, and social media advertising. Make recommendations in accordance with certain marketing campaigns or certain periods of the year. Incorporate eCommerce product recommendations based on the customer’s previous actions in abandoned cart emails to reduce the loss of sales. Customer segments used for marketing should also be applied in recommendation strategies to avoid confusion among customers.
Cross-Selling and Upselling Tactics
Understand what other products customers are likely to be interested in and group them into packages that can be sold together. Utilize past data to recommend a more expensive product or version. Use the cross-sell sections on the product pages. Provide options to upsell during the check out process. Appeal to the social proof by pointing out the most popular pairings that other clients have chosen, under “Frequently Bought Together”. Make sure that cross-sell and upsell suggestions are related to the customer’s planned purchase and would be of benefit to the customer.
Real-Time Recommendation Engines
You can also opt for a specialized product recommendation engine for eCommerce that works in real time, like App0. Provide mechanisms that automatically alter recommendations as soon as the current user behavior and inventory data are available. Employ machine learning techniques to analyze and respond to data as soon as possible. It is possible to use such parameters as the current session, the last purchases, and popular items. Make changes in recommendations based on events that are happening in real-time, for example, flash sales or limited-time offers. Make sure that your architecture is capable of processing data in real time to provide the best user experience.
Top 5 eCommerce Product Recommendations Examples: How to Integrate Product Recommendations Organically?
Integrating eCommerce product recommendations seamlessly into the user experience is crucial for their effectiveness. Here are four key areas where eCommerce businesses can incorporate recommendations, along with real-world examples of successful implementation:
Homepage Recommendations
Homepage banners and other product recommendations are capable of presenting the visitors with specific items once they enter the website. For instance, Stitch Fix, which is an online styling service, has a ‘Shop your looks’ link which shows clothes according to the preference of the user and the previous orders. It helps the users discover new styles that they wouldn’t have normally gone for and makes the purchases.

Product Detail Page Recommendations
Cross selling is enhanced by giving suggestions of other products that can be bought alongside the product in question. An example of a good use of recommendation is Beer Hawk, where on the product pages there is a section called ‘You Might Also Like’ which offers the customer products similar to the ones they are currently browsing. This strategy not only increases the probability of finding the correct type of product, but also increases the chances of customers’ exposure to more products offered by the company.

Shopping Cart Recommendations
Products that are related to the add-ons should be useful and add value to the order when the client is at the checkout point. Dollar Shave Club has a ‘Want More?’ section on the cart page where based on the items in the cart they suggest other grooming products. This strategy makes the customer think about enhancing their grooming routine with special products and thus, increases average order value.
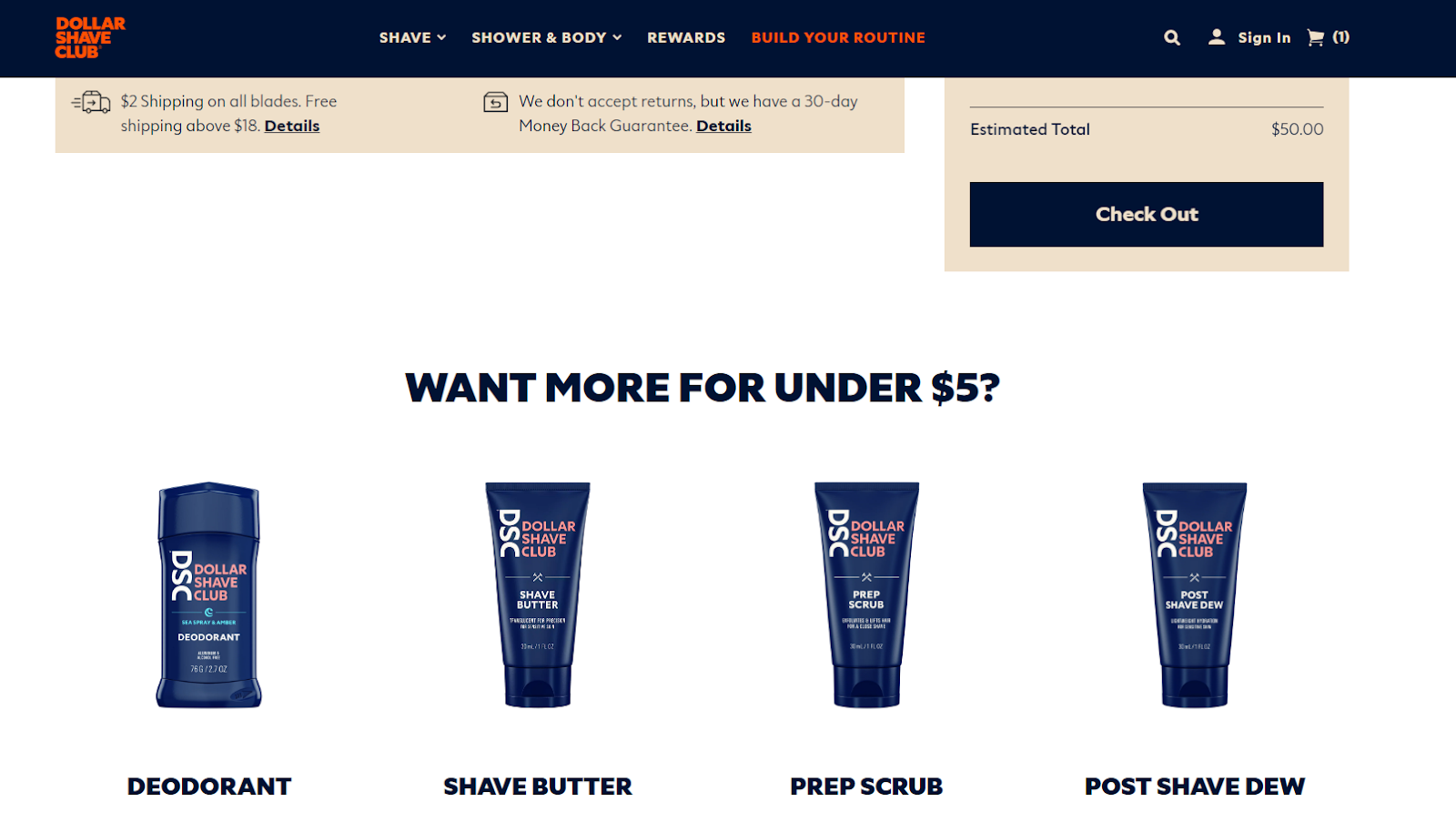
Email Marketing Recommendations
Recommendation through email can influence the customer and can recall them to the site along with the products which they may be interested in. Glossier, a beauty company, sends in informational email campaigns that educate users on creating their own skincare routine - while smartly plugging in their products, and providing flexibility to the buyer. This specific approach ensures that they are always linked uniquely to their products; it also makes their marketing communications more relevant hence enhancing the rates of conversion.
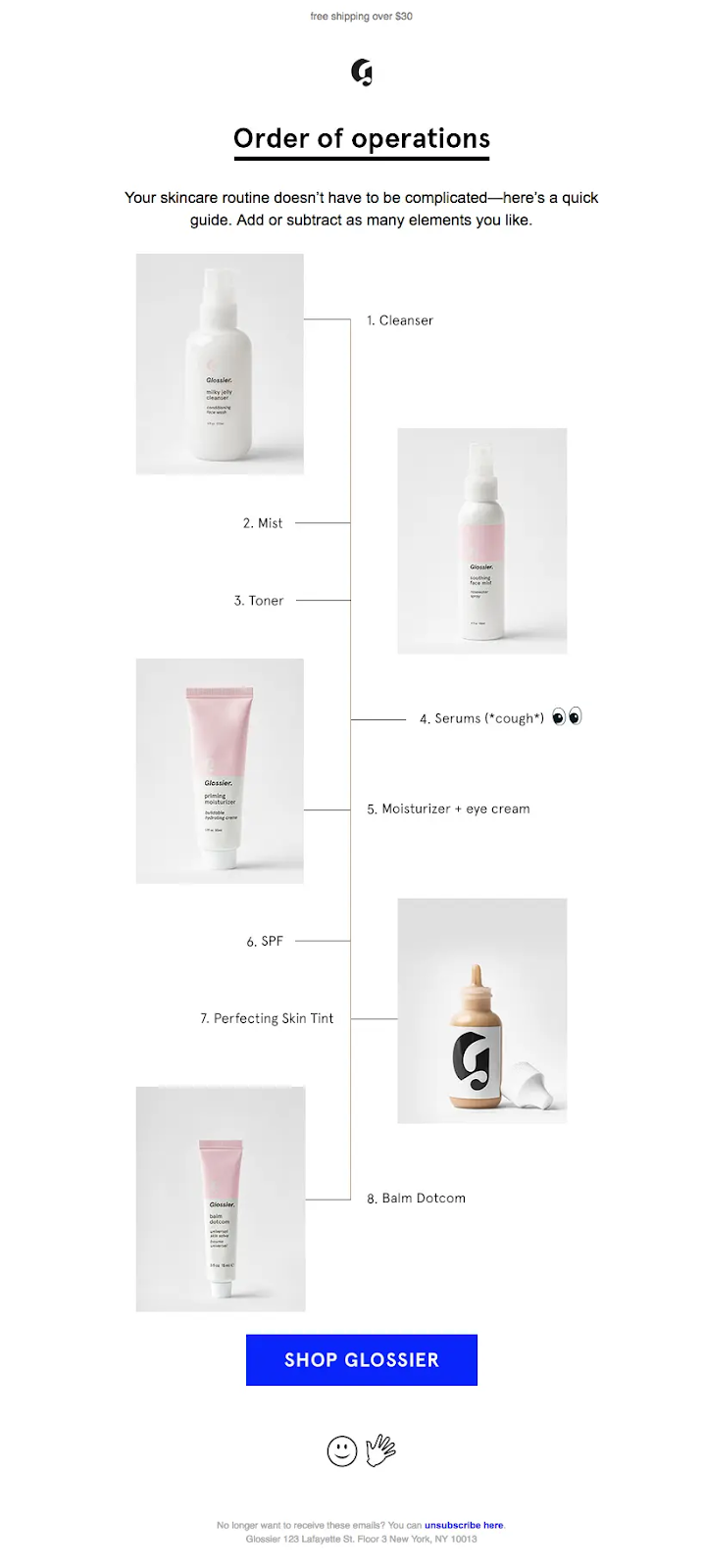
Conversational Product Recommendations
In a way, this technique is an evolved version of the previously mentioned Product Recommendation examples - and certainly the most effective. App0’s conversational AI can push the most relevant eCommerce product recommendations while in direct conversation with your customer - just as a seasoned sales representative does in a physical retail store. Your customers can then ask follow up questions or queries and get accurate responses, making their buying experience seamlessly enjoyable.

Conclusion: Best eCommerce Product Recommendation Engine in 2024
With the onset of Artificial Intelligence. eCommerce Recommendation Engines are becoming increasingly more efficient in 2024 than ever. By leveraging AI and machine learning algorithms to derive concise insights and push the most accurate recommendations, these product recommendation systems excel in achieving the intended effect. But what if you could enhance your AI driven eCommerce Recommendation Engine with even more capabilities?
App0 does more than just push product recommendations - it learns your customers’ buying behaviors and responds to any of their questions in natural language via chat, right at your online storefront.
Personal shopper at your customers’ fingertips
Bring Your Store to Your Customers' Fingertips. Our AI-based personal shopper allows customers to shop as easily as they can chat. Nothing to download, no account or login required.
AI-based shopper tailored to each customer’s needs
AI-based concierge learns the purchase behavior and preferences of your customer to meet their unique shopping needs
Answer any question, Process any request
An AI-based concierge seamlessly chats with your customers to answer any questions, assist with tracking orders, or process returns
- Order tracking
- Return and Exchange
- Product questions
FAQs